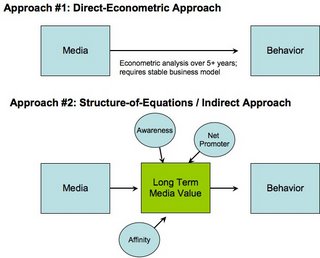
I've had a lot of conversations about with B2B marketers about how to value the long-term impacts of marketing communications. It's easy when you're looking at direct marketing, like email. We can look at the customer level for return data, applying CLV (customer lifetime value), and we can be pretty assured that we have an accurate statement of ROI. When you're talking about above-the-line media (TV, Radio, etc.), though, you have a tougher problem to solve. What I've seen is that most (95%) of media mix models done by companies, agencies, and specialty consulting firms tell you the impact of advertising for a maximum of about two quarters. Past this, causal effects blend in with the error term and we can't see the signal anymore. The trouble is, most marketers will tell you that above-the-line is bought for two reasons: to increase same period sales and to make customers like you more / think of you more over the long run.
Brand value models have been in use for a long time. Interbrand publishes the "Top 100 most valuable brands" every year. Its methodology is pretty top-down. Essentially they start with total revenue for the company and then subtract out stuff that isn't brand related. From this they just NPV "brand related" revenues and hit sort--voila.
This is a great methodology but doesn't really work for advertising optimization because you're not interested in the total brand value, only the part that was generated by media. So, you're back to a statistical methodology that attempts to link an independent variable (advertising spend, media weight, etc.) with a dependent variables (revenues or profits). There are two general ways to do this that I've seen.
1. Econometric-Direct Approach. This is my name for it. Essentially you're getting very long sets of time series data, many years in fact, and attempting to parse out the effect of media on the dependent variables using very long lag periods. There have been several studies done in academia on this front. A good sense of the debate around this issue can be found here.
Benefits: No latent variables required. No sampling of attitudes required. No multi-level analysis (or structured system of equations) required for analysis.
Hazards: Lots and lots of data required. Could be impossible to tease out long-term effects in a dynamic (or "chunky" market--such as software). I'm still not convinced it works at all.
2. Stucture of Equations-Indirect Approach. In this approach, which I prefer, you look at two relationships: First between media and an attitude (or set of attitudes) such as awareness, comprehension, or affinity, and then between these attitudes and customer behavior. Essentially, you're using the attitudes to indicate the latent variable "long run advertising value" in a structural equation model. The nice thing is that attitudes can be sampled easily over the long run (many companies are already doing this), giving good explicit indicators for the latent variable.
To successfully start modeling long-term media value using approach #2 above, you need patience, a good long-term attitude tracker study with DMA cross-sections, and the willingness to experiment some between DMAs. These data can also be supplemented with conjoint-type market research.
No comments:
Post a Comment